The Ultimate Guide to Data Labeling Tools for Businesses
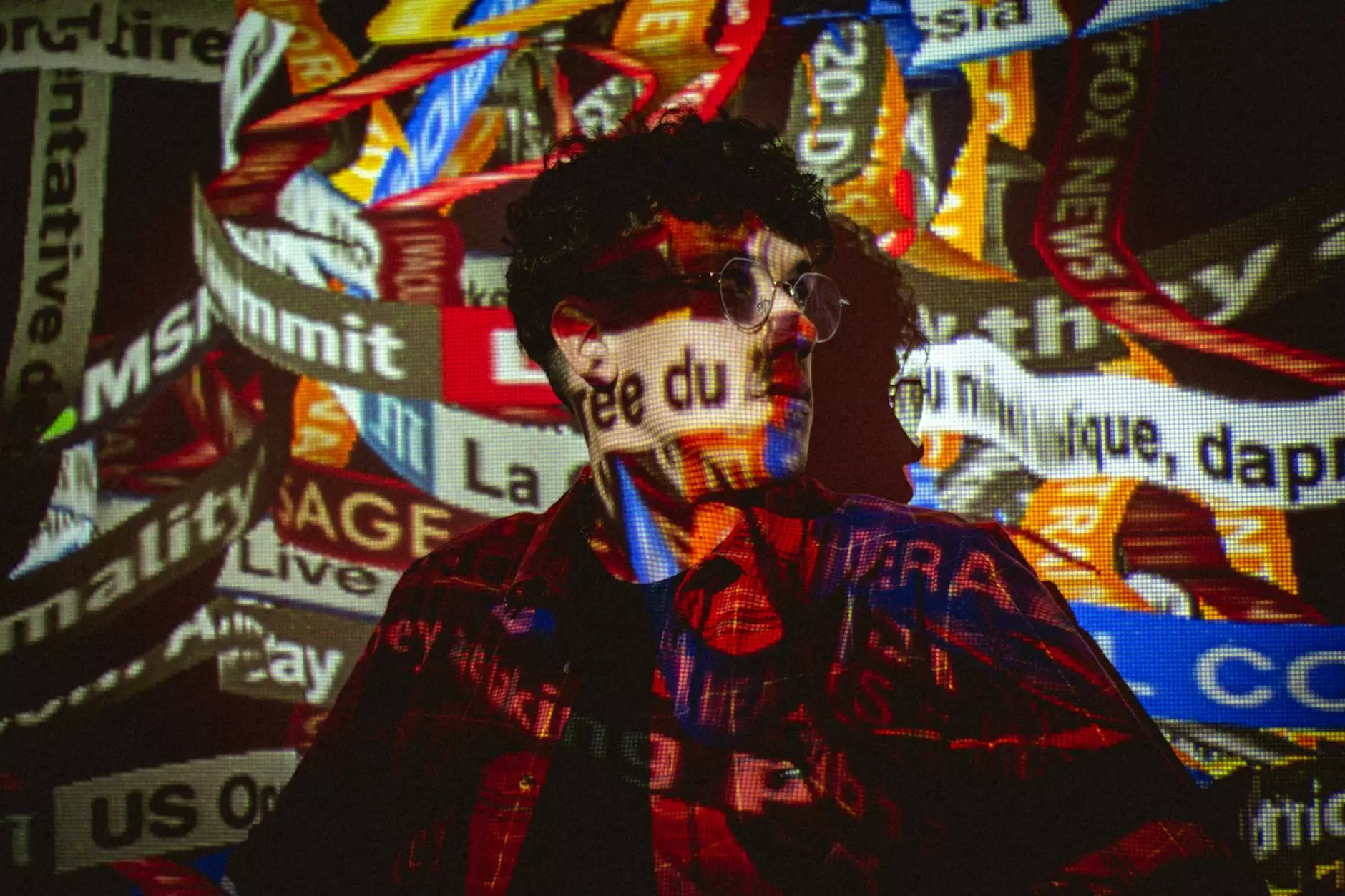
In the rapidly evolving landscape of technology, businesses are increasingly relying on data to drive their decisions and enhance their services. One significant aspect of leveraging data is the process of data labeling, which is crucial for training machine learning models. A data labeling tool can be a game changer for organizations looking to harness the power of data effectively. In this comprehensive article, we will delve into the essentials of data labeling, explore various tools available, and discuss the best practices to maximize the utility of these tools in your business.
Understanding Data Labeling
Data labeling is the process of annotating data to make it understandable for machine learning algorithms. It involves tagging various elements in datasets, including images, text, audio, or video, ensuring that the machine learning model learns from well-defined examples. This process is integral to supervised learning, where the model needs labeled data to make predictions.
Why is Data Labeling Important?
Data labeling is vital for several reasons:
- Improves Model Accuracy: Accurate labels lead to better model predictions. The more precise the data, the more effective the machine learning model becomes.
- Facilitates Advanced Analytics: Labeled data allows businesses to extract deeper insights through advanced analytics, helping them make informed decisions.
- Enhances User Experience: By utilizing labeled data, businesses can tailor their services to meet customer needs more effectively.
- Boosts Automation: Well-labeled datasets enable automation of various business processes, increasing efficiency and reducing human error.
What is a Data Labeling Tool?
A data labeling tool is a software application that helps in the process of annotating data. These tools are designed to streamline data annotation tasks, enabling users to label their datasets quickly and accurately. They provide features such as user-friendly interfaces, collaboration tools, and automation capabilities to enhance productivity.
Key Features of Data Labeling Tools
When selecting a data labeling tool, it’s essential to consider the following features:
- Collaboration Support: Ability for multiple users to work on data labeling tasks simultaneously to enhance efficiency.
- Automated Labeling: AI-driven features to assist in the automatic labeling of data, significantly reducing the time required.
- Integration Capabilities: Compatibility with other tools and platforms to facilitate seamless workflow.
- Customizable Workflows: Options to tailor the labeling process according to specific project requirements.
- Quality Control Mechanisms: Tools for verifying and validating the labels to ensure data quality.
Types of Data Labeling Tools
Data labeling tools come in various forms to cater to different business needs. Here are the main types:
1. Image Annotation Tools
These tools are primarily used for labeling images for computer vision tasks. Common annotations include bounding boxes, polygons, and image segmentation. Popular tools in this category include:
- Labelbox: A comprehensive platform that combines the power of image labeling with high-level collaboration features.
- VGG Image Annotator: An open-source tool for image annotation that supports various shapes and formats.
2. Text Annotation Tools
Text annotation tools are designed for labeling textual data, such as sentences or paragraphs. This can include sentiment analysis, entity recognition, and part-of-speech tagging. Leading tools include:
- Prodigy: An efficient tool for annotating text that employs machine learning to optimize the tagging process.
- Doccano: An open-source annotation tool that supports multiple languages and various labeling tasks.
3. Audio Annotation Tools
For businesses dealing with audio data, audio annotation tools help transcribe and label audio files for speech recognition tasks. Some notable tools are:
- Audacity: A free, open-source platform that can be used for audio recording and editing, including annotation.
- Kaldi: An advanced tool for speech recognition that also includes functionalities for labeling audio data.
4. Video Annotation Tools
Video labeling is essential for tasks such as object detection and action recognition in videos. Top video annotation tools include:
- VideoLAN: An open-source multimedia framework that can be adapted for video annotation and analysis.
- VOTT (Visual Object Tagging Tool): A Microsoft tool that allows users to categorize and tag objects within videos easily.
Choosing the Right Data Labeling Tool for Your Business
Selecting the right data labeling tool for your business requires careful consideration of your specific needs. Here are some factors to keep in mind:
- Project Requirements: Understand the nature of your data and the labeling tasks you need to perform.
- Budget: Evaluate the cost of the tool versus the value it provides to your business.
- Scalability: Choose a tool that can grow with your business and handle increasing volumes of data.
- Support and Community: Ensure there is sufficient documentation and community support for troubleshooting and best practices.
Best Practices for Data Labeling
To ensure optimal results from your data labeling efforts, follow these best practices:
1. Define Clear Guidelines
Establish detailed labeling guidelines to ensure consistency in the annotations. Provide examples of correct and incorrect labels to your annotators to reduce ambiguity.
2. Use Quality Control Processes
Implement quality control mechanisms to regularly check the accuracy of labeled data. This can include peer reviews or employing automated checks to identify common errors.
3. Invest in Training for Annotators
Providing training to annotators on the specific data types and labeling tasks will significantly enhance the quality of the annotations. Ensure that they understand the importance of their role in the machine learning pipeline.
4. Start with Small Datasets
When testing new labeling processes or tools, start with smaller datasets. This will help you fine-tune the processes before scaling up.
Future Trends in Data Labeling
The field of data labeling is continuously evolving with advancements in technology. Here are some future trends to watch:
- Increased Automation: More sophisticated AI algorithms will assist with data labeling tasks, reducing the manual effort required.
- Use of Synthetic Data: Generating synthetic data will become more prominent, allowing businesses to create large volumes of labeled data quickly.
- Real-time Collaboration Tools: Enhanced tools that facilitate real-time collaboration among annotators will improve efficiency and consistency.
- Integration with Machine Learning Pipelines: Data labeling tools will increasingly be integrated with machine learning platforms, allowing for seamless transitions from data preparation to modeling.
Conclusion
In conclusion, adopting an effective data labeling tool is essential for businesses that wish to leverage the power of data for machine learning and artificial intelligence applications. By understanding the importance of data labeling, selecting the right tools, and following best practices, organizations can enhance their data capabilities, drive meaningful insights, and ultimately achieve a competitive edge in the market.
To explore leading data annotation tools that can transform your data labeling processes, visit keylabs.ai and discover how our solutions can elevate your business to new heights.